
Capstone

CHAPTER 4
Results and Discussion
This study employed the novel workflow of Briones et al. (2021) to investigate the interaction between phytochemicals present in Vitex negundo and proteins associated with psoriasis. The study aimed to explore the potential of Vitex negundo in treating psoriasis and provide insights into the underlying mechanisms.
1 / Phytochemical Compilation of Vitex Negundo
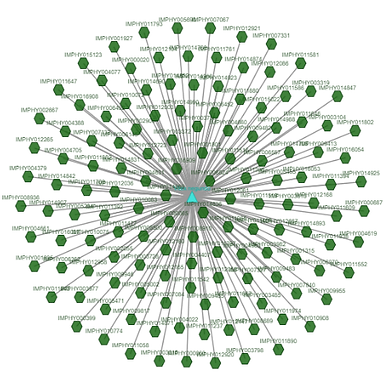
The plant Vitex negundo, as recorded in the IMPPAT database, yielded a total of 48 phytochemicals. Among these phytochemicals, the majority were categorized as prenol lipids, flavonoids, stilbenes, cinnamaldehydes, fatty acyls, and drugs.
​
Several studies reviewed in the research identified specific prescribed drugs that exhibit inhibitory effects on disease proteins within the TNF pathway. These drugs, which are considered the positive controls for this study, include Idelalisib, Bortezomib, Thalidomide, Celecoxib, Ibuprofen, Resveratrol, Acetylsalicylic acid, Alpelisib, Wortmannin, IC87114, Asiatic Acid, GSK690693, Capivasertib, PG-116800, Batimastat, Prinomastat, Vinfosiltine, Umbralisib, and VX-745. These drugs have been previously studied and are recognized for their inhibitory effects on proteins associated with the TNF pathway.
2 / Reverse Screening

The compiled list of phytochemicals from Vitex negundo and the positive controls were subjected to analysis using the SwissTargetPrediction tool. This analysis revealed a total of 2011 predicted potential protein-chemical interactions (PCPIs) with nonzero probabilities between the 48 phytochemicals and 25 target proteins.
To perform the analysis, the PCPI list was inputted into the SwissTargetPrediction tool using the SMILES code representation of the chemicals. The chosen category for the analysis was Homo sapiens, indicating that the predictions were specifically focused on interactions within the human organism. The results generated from this analysis included the target protein, target class, CheEMBL ID, and UniProt ID of the 2011 phytochemicals, all accompanied by nonzero probability scores.
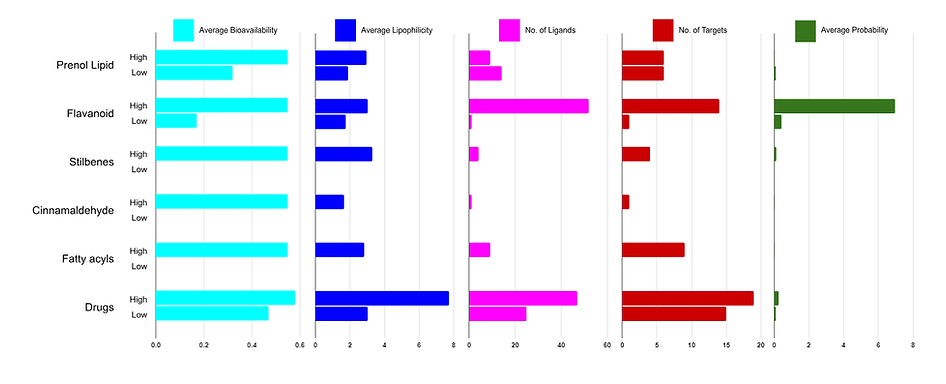

3 / Network Building
An analysis was conducted using g:Profiler and the KEGG database to study a list of target proteins. The results revealed 624 enriched pathways, with the tumor necrosis factor (TNF) pathway identified as significant for psoriasis. Flavonoids, a class of polyphenolic plant compounds, showed the highest number of target proteins and demonstrated potential for rapid gastrointestinal absorption. However, established drugs still showed promise in terms of safety and efficacy, surpassing flavonoids in these aspects. Protein kinases, specifically MAPK8, MAPK10, MAPK3, MAPK9, MAPK14, CHUK, and AKT1, were found to have the highest number of targeted proteins, with proteases being the most prevalent ligands.
​
The relationship between SwissADME parameters and SwissTargetPrediction results was depicted in a figure, showcasing the influence of bioavailability, lipophilicity, and gastrointestinal absorption on ligand classes. The positive control, representing established drugs, displayed higher average bioavailability and lipophilicity, indicating their effectiveness in systemic circulation and absorption. Stilbenes exhibited the highest lipophilicity among the ligand classes, while flavonoids showed the highest gastrointestinal absorption potential. The figure also provided insights into the number of proteins per protein class, the phytochemical ligands targeting each class, and the average probability of targeting cytokines, which exhibited the highest likelihood of being targeted.
4 / Evaluation via Molecular Docking
Table 1: Descriptive statistics of docking scores for drugs
Parameters | Docking Interaction Energy (kcal/mol) |
---|---|
Lower bound | -10.6 |
Upper bound (hard cutoff) | -4.0 |
99.7% Confidence (3*STDEV.P) | 3.29541411 |
STDEV.P | 1.098483804 |
Mean | -7.3 |
Lowest value (soft cutoff) | -6.5 |
Table 2: Descriptive statistics of docking scores for phytochemicals in reference to docking scores bracket of drugs
High Confidence ( x < -6.5)
Parameters | Probability |
---|---|
Upper bound | 7.8 |
68% Confidence interval | 1.227678165 |
Sample Size | 23 |
STDEV.P | 0.6138390823 |
Mean | -7.9 |
Middle Confidence ( -6.5 < x > -4.0)
Parameters | Probability |
---|---|
STDEV.P | 0.6965062582 |
Sample Size | 32 |
68% Confidence interval | 1.393012516 |
Upper bound | -6.3 |
Lower bound | -6.4 |
Mean | -6.4 |
Low Confidence ( x < -4.0)
Parameters | Probability |
---|---|
STDEV.P | 0 |
Sample Size | 1 |
68% Confidence interval | 0 |
Upper bound | -3.9 |
Lower bound | -3.9 |
Mean | -3.9 |
Molecular docking, performed using AutoDock Vina, was employed to model the interaction between small molecules and target proteins. This in silico method allowed for the characterization of the behavior of small molecules in protein binding sites. The docking scores of the drugs and phytochemicals from Vitex negundo (lagundi) were analyzed statistically to compare their interactions with target proteins. The drugs' docking scores served as references for assigning confidence levels to the lagundi phytochemical-protein interactions.
The researchers calculated the upper and lower boundaries for lagundi phytochemical-protein interactions based on the docking scores of the drugs. The least negative docking interaction energy among the drugs was considered the "soft cutoff," while the upper bound of the 99.7% confidence interval was defined as the "hard cutoff." Interactions were then classified into three confidence levels: "High docking confidence" for interactions with docking scores lower than the soft cutoff, "Medium docking confidence" for scores between the soft and hard cutoffs, and "Low docking confidence" for scores above the hard cutoff.
Among the 57 docked interactions, 25 were classified as having "High confidence level." Interactions involving the flavonoids class mostly exhibited high docking confidence, while the interaction involving the sole stilbenes compound had low docking confidence. This analysis provided insights into the quality and reliability of the lagundi phytochemical-protein interactions based on their docking scores and confidence levels.
Table 3: Student’s t-test between means of phytochemical and drugs
Parameters | Phytochemical | Drugs |
---|---|---|
Mean | -7.771428571 | -7.766666667 |
Variance | 1.040659341 | 1.010666667 |
Observations | 14 | 6 |
Hypothesized Mean Difference | 0 | |
df | 10 | |
t Stat | -0.00966444 | |
P(T<=t) one-tail | 0.49623955 | |
t Critical one-tail | 1.812461123 | |
P(T<=t) two-tail | 0.992479099 | |
t Critical two-tail | 2.228138852 |
5 / Docking Evaluation through Visualization
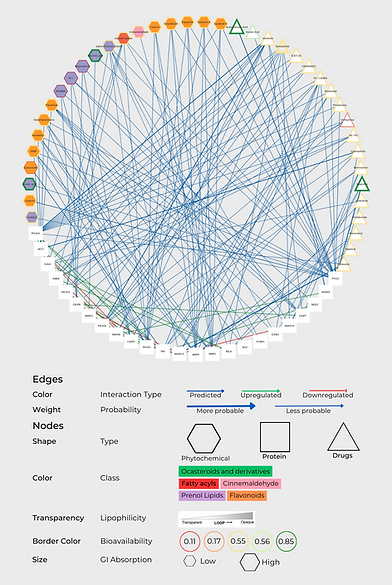
The binding affinity between the ligands and receptors was visualized in 3D using UCSF Chimera, providing insights into the interactions and comparing them with the controls. However, due to the lack of in silico data for the specific disease, the researchers extracted relevant data from previous studies to map the interactions using Ligplot. The tabulated docking scores for lagundi phytochemical-protein interactions and the determined positives (drugs with inhibitory effects) aligned with prior research on psoriasis treatment targeting the TNF signaling pathway.
Among the proteins targeted by the phytochemicals and drugs, PTGS2 (COX-2) emerged as the major receptor, with the highest number of interactions. PTGS2 is involved in the inflammatory response and is dysregulated in psoriasis. Its upregulation leads to increased production of prostaglandins, contributing to inflammation and abnormal skin cell growth. In psoriasis, COX-2 expression is influenced by cytokines such as TNF-alpha, IL-1, and IL-17. Targeting COX-2 and its products, such as prostaglandins, has been explored as a potential therapeutic approach for psoriasis, with nonsteroidal anti-inflammatory drugs, including selective COX-2 inhibitors, used to manage symptoms.
However, psoriasis is a complex disease involving immune cells, inflammatory mediators, and skin cells. While COX-2 and prostaglandins play a role, there are other pathways and factors involved. Therefore, treatments for psoriasis often involve a combination of approaches to target different aspects of the disease, including immune modulation and managing skin cell growth.